Annika Van Vlack, Hui Wong, Claire Lin
Our data story can be found here: https://sites.google.com/view/info419finalproject/home
The objectives
Our chief objective, as spoken to in our data story, was to explore housing data in Vancouver through visualizations that could make trends apparent. We did not intend to construct an argument prior to exploring the visualization, and neither we do not want to present a clear thesis based on the visualization. In terms of actions through Tamara Munzner’s (2015) scheme, we had to first visualize data to support our own high level action of “discovery,” middle-level action of “exploration,” and query the data as it came (p. 46).
We present a survey of trends that we found to be of note, not a thesis or conclusion. We wanted to stress this point in our data story because our data lacks context that may help point to causal factors behind trends—as data often does.
We intend to allow users to casually encounter and “enjoy” the data, as a high level action (Munzner 2015, p. 48).We want to mirror such encounters and enjoyment of the data with our own exploration, and thus include interactive components in our data visualizations that would support middle- and low- level search and query goals. In doing so, we hope that users will be able to engage closely with what we found to be worth presenting, forming a more comprehensive picture of housing in Vancouver.
The data set
We used the Canadian census dataset, taken once every five years, and the 2011 National Household Survey dataset (see bibliographic references for links to these data sets). These datasets are available through open data portals from the city of Vancouver and Statistics Canada. These datasets prove to have a few benefits: first, they are credible and valid data with clear provenance. They are collected directly from government censuses. Further, both the city of Vancouver and Statistics Canada provided notes on data accuracy, including rationale for data rounding and possible omissions. These censuses have a high response rate and count. These datasets are complex and contain a lot of information that we sifted through and pared down.
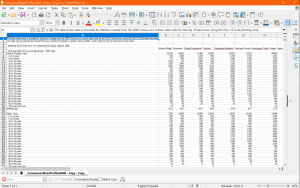
The 2006 census data
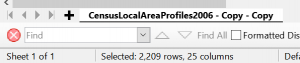
2209 rows! 25 columns!
We used LibreOffice Calc, Microsoft Excel, and Tableau Prep to clean the data, as well as put them into single data tables ready to be read by Tableau. By the end of it, we had two cleaned data tables: one for data of Vancouver’s neighborhoods from 2006-2016, and the other for data about Vancouver at large from 2006-2021. The reason for this is because the 2011 census did not take comprehensive neighborhood data about certain things like incomes; as well, the 2021 census is not split into neighborhoods. We negotiated this in our data story by focusing on neighborhood data separate from Vancouver-wide data.

Sample of cleaned dataset
We did not modify the data too much, save for adjusting for inflation to make incomes consistent, and deriving data to find things like rates of changes and differences between years.
Lastly, for our geospatial dataset, a .geojson file provided by the City of Vancouver was available with the census. This file required minor modifications to move marks on visualizations to appropriate locations.
The tools
Microsoft Excel/LibreOffice Calc/Tableau Prep: These were our data cleaning tools. We used our spreadsheet tools because of their multifaceted strength in cleaning data by supporting simple functions like search and replace, deleting rows, and deriving data through formulas. Tableau Prep was the simplest way to merge sheets and ensure that something would be ready for Tableau, the chief information visualization tool that we would be using. These tools strengths are that they are simple, reliable, and fairly accessible. A possible weakness may have been that they struggled when we tried to use especially large datasets, common with comprehensive censuses. Thankfully, these datasets ended up not being used.
Bank of Canada Inflation Calculator: Since a large part of our project was focused on looking at financial data through time, we needed to account for inflation. During the data cleaning process, we used the Bank of Canada’s Inflation Calculator to make our monetary data appropriately consistent for comparison.
Tableau Desktop/Online/Public: Tableau was key and central to our project. We were most comfortable with this tool as it was what we had learned from class; our use of Tableau informed our conceptual knowledge of data visualization, too. It was through using the tool often that we learned how best to apply theoretical ideas about producing effective visualizations Perhaps the most important strength, however, was Tableau’s ability to provide interactive features in embedded links, something that other tools like Canva and Infogram did not afford. On the other hand, a weakness of Tableau (as opposed to something like Infogram) is that it takes more technical knowledge to make visualizations appealing or “beautiful”, something Lankow et al. (2012) suggest might be taken into consideration when designing public-facing infographics.
After we created our visualizations, we had to figure out how to embed them in our Google Site (discussed later) using Tableau Public. The process was simple and largely intuitive, if somewhat glitchy. One of the features of Tableau Public that we appreciated the most was the online editing feature, which allowed us to edit our published, embedded dashboards without needing to delete/re-embed any visualizations. This proved helpful late in the process when the site was all formatted but we realized we wanted to change colors, tooltips, annotations, etc. There were certainly roadblocks, however; we were met with a stubborn error message while trying to upload. While never fully resolved, we were able to proceed with a creative workaround. Later, as helpful as we found the live editing feature, some edits we made on Tableau Public’s online editor did not publish properly or did not translate properly to their embedded versions. While Tableau Public proved one of the most technically frustrating parts of process, the results–fully interactive embedded visualizations–were worth the effort.
Google Sites: Google Sites served our purpose well. Initially, we tried to use WordPress, but the formatting and “blocks” style proved too fiddly. Additionally, it did not easily support collaborative work. Once we switched to Google Sites, it was smooth sailing. Embedding visualizations from Tableau Public was easy, and while there was some formatting difficulty, it was minimal. Another aspect of Google Sites that served us well was the fact that multiple group members could view and edit the site before it was published.
The analytic steps
The only thing we had agreed upon at the start was a focus on Vancouver. We had an eye to our audience, who were likely to be familiar with the city. We initially wanted to focus on the impacts of Covid-19 across 2016-2021. This focus was born out of exploring a number of datasets on the City of Vancouver’s open data portal, and finding potentially generative information in the 2016 census dataset. However, we came to find out that the 2021 dataset had not yet released all of its information available, which meant there would simply not be enough meaningful data to visualize. We therefore pivoted to a longer-scale data story from 2006 to 2021, choosing something else that was pertinent to Vancouver: housing and living costs.
Because of the nature of data, we were still wary of presenting arguments, which may point to causal factors, based on trends and correlations. Therefore, with the idea that we would be creating visualizations for what Munzner (2015) terms “discovery” and “exploration,” we took steps that would leave a lot of attributes in our cleaned data. Because census data is so large and messy, we still had to remove a lot of data; nonetheless, we kept much of the data that related to housing and affordability. Then, we explored visual idioms with that data, noting down what were interesting trends.
Our data story is thus a reflection of this data exploration process. Our hope is for users and readers to explore the data as we did, as well as “enjoy” consuming it (Munzner 2015).
The design process and principles
We held in mind Munzner’s design principles of expressiveness and effectiveness–the idea that information should be visualized in a way that expresses data attribute types in their appropriate channel (expressiveness) and that information visualization should make salient its most important elements–as well as ethical design principles as discussed by Jonathan Schwabish and Alice Feng (2021). Schwabish and Feng’s ideas about asking certain questions to the data (such as “Who is included and who is excluded from these data?” [p. 11]) are crucial to a sensitive topic like ours, and our data story centers ethical design by paying attention to the ways in which housing in Vancouver can give a more comprehensive picture of that which is underrepresented.
Following Lankow et al. (2012), we tried to strike a balance between retention and comprehension, both crucial parts of utility (p. 199), by our use of familiar idioms and narrative techniques while attempting to ensure our data does not mislead to deceive. Where Tableau afforded, we provided as much aesthetic appeal as possible with formatting and fonts; we matched the blue theme of the images of Vancouver that we used. Lastly, we believe our data story fufils the call for what Lankow et al. (2012) suggest is a crucial part of “soundness”: a data story should “communicate something meaningful” (p. 200).
While including interactions into our visualizations was always on our minds, when we began embedding visualizations into the site we realized that using dashboards with multiple visualizations was essential. While this originated as a choice made because of formatting, it directed us to think more deeply about our use of interactions. While we already had employed many highlighting and filtering actions, in our creation of dashboards we intentionally incorporated brushing and linking (Heer and Shneiderman 2012, p. 13). As well as offering a more advanced, integrated form of interaction, this addition also prompted us to consider how our visualizations related to one another, and how linking them via interactions could reveal connections and help the data tell a story.

An area chart, which we deemed ineffective and replaced with a tree map in our data story.
For geospatial data, the expressiveness principle is achieved because spatial data is visualized using spatial channels, and both size and saturation used to express quantitative variables (choropleth and symbol maps). Based on Munzner’s (2015, p. 102) scheme for ranks of effectiveness, the spatial channel, which is most effective, is already taken by the map data. This is something Munzner acknowledges (p. 180). Then, we chose to use saturation to encode quantitative attributes for many of the maps, and symbol maps with choropleth maps to encode a comparison of two different attributes. Though area is, in Munzner’s scheme, more effective than saturation, we decided that the widely-used choropleth map, with our Vancouver-blue color scheme, for most of our visualizations would be more familiar and more appealing, both of which Lankow et al. (2012) take to be important. Thus, we believe that our geospatial visualizations are effective as they nonetheless pay attention to preattentive features, as well as use annotations to make information more salient. We centered data that focused on income divisions and housing density to emphasize the ethical element of our project, wishing to give some insight into how trends surrounding housing affect different individuals differently.
The story
We wanted to help users and readers gain a more comprehensive picture of housing in Vancouver beyond platitudes like “everything is getting worse” or “we just need the market to bounce back.” Beyond questions of economics, we wanted to center our visualization on people who live in Vancouver, and what trends may be able to shine light on regardingtheir diversity. Therefore, our story comes in the form of questions that we decided to ask: questions about one-parent households, affordability, household sizes, population and income, and spread of dwelling types take precedence. We want to guide users of our data story with captions below our infographics, but also allow users to explore the data on their own with interactive features, just as we had.
If there is an argument our views may be said to support, it would be that over-simplifying Vancouver’s housing market to absolutely good or bad is a mistake. Views demonstrate that the relative (un)affordability of single-households in Vancouver has been true for a long time in Vancouver, while also showing the way different neighborhoods are differently affected. The story we want to tell is one of complexity: data oftentimes complicates, not simplifies, and this is a good thing.
The pros and cons
The reason the idiomatic “data is never raw” or “all data is cooked” is so often repeated is that data as such commands a certain authority–numbers cannot be wrong. There is a certain intuitive wish to take data as end-all-be-all, and no matter how much we might have considered “soundness” or “utility” and Munzner’s design principles, wrangling data is difficult.
We strongly believe that telling a story with data can be effective. In our data story, we attempt to emphasize the fact that though we identify trends, data requires context. A strength of data is that it can buttress points made about our ethical relationships to the world and, in our case, to housing, the economy, and Vancouver. Data, we suggest, is at its best when used as one part of a holistic picture, and used in such a way that encourages exploration to learn with the data. So perhaps a strong part of our designs is the use of contextual information in captions, and the interactivity that allows data exploration.
Some logistical cons of our data include the possibility of human error when it comes to cleaning large datasets, the question of whether our visualizations may be inconsistent across screens and screen colors, and the possibility that comes with geospatial data that hue and saturation over wide areas may be more difficult to discern. Outside of logistics, cons of working with data include the possibility, and the lull, of using data to leap to illogical conclusions without sufficient contextual evidence.
Nonetheless, data can be potent as an aid to a larger, more complex, story.
Other Links
Google Sites (main deliverable): https://sites.google.com/view/info419finalproject/home
Tableau Public with all visualizations: https://public.tableau.com/app/profile/annika7277
Bibliography
Census local area profiles 2006. (March 25, 2013). City of Vancouver. Retrieved October 19, 2022, https://opendata.vancouver.ca/explore/dataset/census-local-area-profiles-2006/information/.
Census local area profiles 2011. (November 13, 2013). City of Vancouver. Retrieved October 19, 2022, https://opendata.vancouver.ca/explore/dataset/census-local-area-profiles-2011/information/.
Census local area profiles 2016. (April 10, 2018). City of Vancouver. Retrieved October 19, 2022, https://opendata.vancouver.ca/explore/dataset/census-local-area-profiles-2016/information/.
Census Profile, 2021 Census of Population. (2022). Statistics Canada. Retrieved October 19, 2022. https://www12.statcan.gc.ca/census-recensement/2021/dp-pd/prof/details/page.cfm?Lang=E&GENDERlist=1,2,3&STATISTIClist=1&HEADERlist=0&DGUIDlist=2021A00055915022&SearchText=vancouver.
Heer, J., & Shneiderman, B. (2012). Interactive dynamics for visual analysis: A taxonomy of tools that support the fluent and flexible use of visualizations. ACM Queue, 10(2), 30-55. https://doi.org/10.1145/2133416.2146416
Lankow, J., Ritchie, J., Crooks, R. (2012). Infographics: The power of visual storytelling. John Wiley & Sons, Inc.
Munzner, T., Maguire, E., & CRC Press. (2015). Visualization analysis & design. CRC Press, Taylor & Francis Group. Ebook Version.
NHS Profile, Vancouver, 2011. (2011). Statistics Canada. Retrieved October 19, 2022. https://www12.statcan.gc.ca/nhs-enm/2011/dp-pd/prof/details/page.cfm?Lang=E&Geo1=CMA&Code1=933&Data=Count&SearchText=933&SearchType=Begins&SearchPR=01&A1=All&B1=All&Custom=&TABID=3.
Schwabish, Jonathan and Alice Feng. (2021). Do No Harm Guide: Applying Equity Awareness in Data Visualization. Urban Institute. https://www.urban.org/research/publication/do-no-harm-guide-applying-equity-awareness-data-visualization.
Hi guys! Wonderful work on the visualizations! It looks like you’ve spent a lot of time and effort on this. Well done!
I loved how you chose not to construct an argument before exploring the InfoVis yourself — I think it allowed you to be as unbiased as possible. Your data seems solid and credible. So as a user, I have no problem trusting a visualization built on this data. Also, it looks like you’ve given your design process a lot of thought, so this design is very well theory-supported.
The visualizations I enjoyed the most were “income by neighbourhood” and “explore by neighbourhood”. Interactive features allowed me to filter out data I wasn’t interested in at the moment and easy explore the one I was interested in.
What made it a bit difficult to understand your InfoVis, is the size of circles in the “household size” visualization. I might be just me, but it was hard to differentiate the sizes of circles. For me, $26.000 circle and $40.000 circle looked a bit the same. Also, it might be just me again, but I couldn’t find the after-tax values for 2011.
For “what kinds of dwellings do Vancouverites live in”, I was associating the position of each segment with percentage of people living in this particular building type. So, in my head, almost 100% live in an apartment, and I am pretty sure this is not quite correct.
All in all, it a great job! I enjoyed exploring your project a lot!
Hi Guys!
It was really interesting to read your article with the data visualizations!
I think this was a really interesting topic that you guys approached with sensitivity. The goal here is not to simply tell Vancouver housing is getting more expensive, but also to shed light on who can afford these regions what are the people that live in these regions. Are they single, families. What is the minimum overall you need to afford living here. I think one thing it would be nice to have, since we are splitting into neighbourhoods is how much it costs to live in each. I guess it correlates to how much people are making but it would still be interesting. For what I saw on the post you guys might have found it not relevant as there is a lot of information regarding the rest. As well, I noticed you guys kinda of separated in blocks what you wanted to focus more in each section. I think you did an amazing, really amazing job in guiding the user on how to explore and make the most out of the graphs. Honestly, really impressive work!
I see that this is a really exploratory work, but specially because of the amount of information it might get a bit overwhelming for one to connect what they learned in view one to the last one. I think I would have more highlights of what I want them to remember later, just like you guys did with downtown Vancouver textbox.
I am really glad I got to see your work 🙂