Voice To Text/Speech Recognition Technologies
Technology is pervasive and inundates every aspect of our lives in Western Society today. Ever since Alan Turing developed the Turing Test in 1950, and Bell Laboratory’s Audrey, humans have been trying to simulate human speech. From the early days of the computer and word processing programs there has been a call for voice recognition software to help increase the flow of thought to page. Speech recognition software uses natural language uttered by humans to a machine to perform an action by a smartphone, computer, or other devices (Technologies, 2022). The technology has humble beginnings in 1773 Russia (Moskvitch, 2022), though it was not officially recognized or successful until 1952, when Audrey arrived at the phone company. She had limited success, however, until the idea caught the attention of the military. With the single-minded purpose of military invention for making war and national defence more effective, the scientists at DARPA created “Harpy”, who had a vocabulary of 1000 words equal to that of a toddler (Technologies, 2022).
The next major leap in speech recognition technology went from the military to the toy factory. In 1982, “Julie” was introduced as the world’s first ‘talking’ doll. She had a chip that recognized speech sounds and could respond to them appropriately (Technologies, 2022).
Up to this point, speech recognition software was based on the smallest unit of sounds within speech (phonemes), so was limited in what it could recognize. It had difficulties with accents, various speakers, or those who did not enunciate clearly.
As demand grew, and this manner of speech recognition was untenable, scientists began to turn to natural language processing (NLP). This system used algorithmic processing to determine what was said, using it’s best guess to determine words it did not understand, based on programmed rules for the language.
This is where speech recognition sat and waited for man’s reach to catch up to ambition. The global corporate giant, Google, introduced the first innovation in speech recognition software over a decade after Julie and Harpy were introduced. As phones became smaller and more portable, typing on them became more challenging. People wanted and needed an easier way to input data than typing it out on a tiny keyboard on their phone. Now Google offered voice-based commands for searching anything. “Google, what is this song called?” “Google, what is the weather today?” have now become common phrases heard in many households.
Interestingly, in a situation of life imitating art (or science fiction, in this case), the inspiration for Amazon’s work on Alexa came from the computer voice in Star Trek.
Speech recognition technology had now come full circle – what started as oral communication centuries ago became written communication, became electronic communication, and is now back to oral communication.
Dictation technology started simply, from a manager talking to a human assistant who took notes word for word in a shorthand language to be later transcribed into full words, to stenographers recording court proceedings by pressing a few buttons in a machine to transcribe to full words, to physicians dictating notes into a phone recording to be transcribed by a human into a written report. These humble beginnings evolved into increasingly sophisticated technologies as the need for increased efficiency, cost savings and short turnaround times increased in the faster paced world of today. “While companies differ in whether the technology is offered as a replacement of medical transcription or as a tool for assisting the process, a consistent claim across the speech recognition industry is that SRTs [speech recognition technology] can reduce costs due to faster turn-around times of medical documentation, higher efficiency, and increased accuracy (David et al., 2009, p. 926 – 927).
Voice-to-Text technologies have become much more popular, particularly for those who have difficulty with written language and writing and this technology has eliminated the need for a trained human to translate or transcribe words from shorthand notes or voice recording. But has it really eliminated this need? David et al (2009) report that human, specially trained medical transcriptionists (MT) are still required. They state: “…the work of MTs is far more complex than just typing what is spoken in voice files. Their work requires complex professionally-informed interpretive acts that in turn require sustained attention to the social order properties and content of the doctor’s dictation, knowledge of medical terms and procedures, and an understanding of interactional processes, conventions of dictating, and of producing monologic speech acts.” (David et al., 2009 p. 925). So, while speech recognition technology is advanced, easy to use, and efficient, in some cases, such as specialized dictation contexts, humans are still required to interpret and edit the produced text. “Although not designed or even conceptualized to benefit students with disabilities, this concept would definitely have an impact on the learning and access to material for students with all different types of disabilities.” (Bakken et al., 2019, p. 51).
What bearing does this have on pedagogy? In nursing and medical education, it can have significant impact.
For post-secondary institutions, technological advancements also benefit students, faculty, and staff alike. Technologies like simulation and virtual reality are used more extensively now in nursing education than ever before, and are, in some cases, replacing actual ‘hands on’ practice on real patients. This technology has become even more commonplace since the pandemic necessitated withdrawing students from clinical practice environments such as hospital units. “Note taking has remained a learning strategy in academic settings since the time of Socrates.” (Emory et al., 2021, p. 235 – 236)
Traditional classrooms, including those in nursing education have relied on students taking their own handwritten or typed notes. “Note taking is a complex cognitive task that requires students to listen, temporarily store information in the short-term memory, paraphrase, and write down the information before losing it, all while attending to incoming new information. Effective note taking requires management of these cognitive demands” (Emory et al., 2021, p. 236)
Even without medical masks in the classroom, students are still barricaded from view of the instructor behind their laptop screens. The laptop and mobile device are now used for note taking in the classroom. It is rare to see pen and paper used for taking notes in any learning environment.
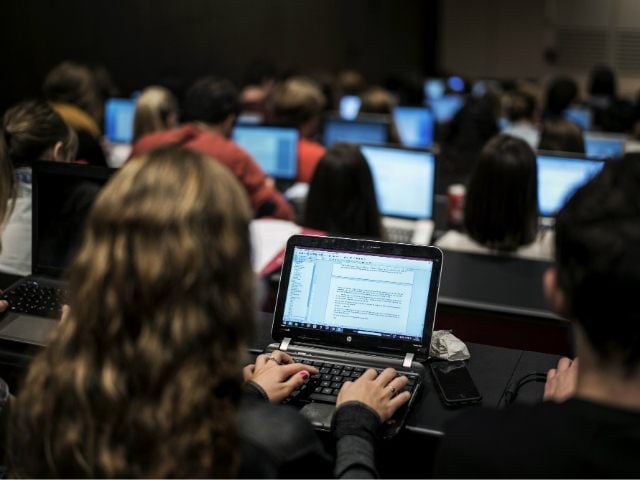
The studies disagree on which is better for learning and retention between handwritten and electronic note taking (Emory et al., 2021). None of the sources reviewed mentioned using voice to text technologies for classroom use, particularly around taking notes. The rise of accommodations in nursing classrooms demonstrate that modern technologies are required to keep pace with demand. Increasingly, students are recording lectures for later transcription and closed captioning through voice recognition is used more often in Zoom and online meetings and classroom presentations. Though this use of real time written transcription during classroom activities can be distracting for some learners, it is valuable to others who may have difficulty keeping up with, understanding or hearing the speaker.
Another possibility for SRT is during the nursing student’s clinical practice. Documentation is a large part of the professional nurse’s job. While electronic health records (EHR) are now the norm in most hospitals and health centres, they vary widely in effectiveness, usability, and comprehensiveness. Currently, most health care settings, along with health care education is verbal and text based. The only time SRT is used is for closed captioning of recorded or live presentations or events and for medical dictation. The uses, however, could be applied to the day-to-day functioning of the nurse and nursing student. It is possible to, for example, record a post clinical meeting (with all participants’ permission) for later review by students. The learning that happens during post clinical meetings is invaluable, with often rich discussions and reflection around actual patient and clinical situations, with guidance from the clinical instructor. These are ample opportunities for consolidation of knowledge, though unlike a lecture or classroom discussion there usually aren’t structured conversations or opportunities for note taking. A transcribed recording of a discussion of a complex patient scenario could serve as study tools for practical exams, such as the nursing graduation exams.

Another scenario where SRT could be utilized is for documentation during a clinical shift. Often, in hospital environments, a student will have multiple patients to provide care for and little time to document that care in between patients. Most often, nurses must save up their data (usually on a piece of paper in their pocket, a ‘cheat sheet’) and enter it into the electronic chart at the end of the shift. While documentation is supposed to be done in ‘real time’, as soon as the event occurs, this is often not possible with the heavy workload demands of nurses and nursing students on a busy hospital unit. If they could carry a hospital tablet or device with them, instead of a piece of paper, they could dictate their notes right away and enter it into the system. Most voice-to-text applications will allow the user to edit on the fly, and depending on the app, will punctuate and edit as well. This could save time, as the nurse would only have to check the already documented notes. This would also help prevent events going undocumented before a major crisis, which can happen as things change quickly in acute situations. A patient can deteriorate before the nurse has had a chance to document, and must record events after the fact, rather than as it is unfolding.
Bringing voice to text technology back to the classroom, it is becoming more prevalent and useful for not just students who are other abled, but all nursing students in a complex and ever-expanding field. “As technology has changed over the last decade, some students report increased preference and usage of electronic devices to augment their learning.” (Emory et al., 2021, p. 243).
In nursing, as with other fields of education, the use of technology is unavoidable and encouraged. “Nurse educators should consider the positive advantages of these devices to actively engage students in the classroom, as many strategies using the latest advances in technology have been implemented with success. Engaging students with these devices can keep them attracted to the classroom activities. The use of advances in technology is critical to nursing program to prepare students in the uses of technology for the jobs of the future.” (Emory et al., 2021, p. 243).
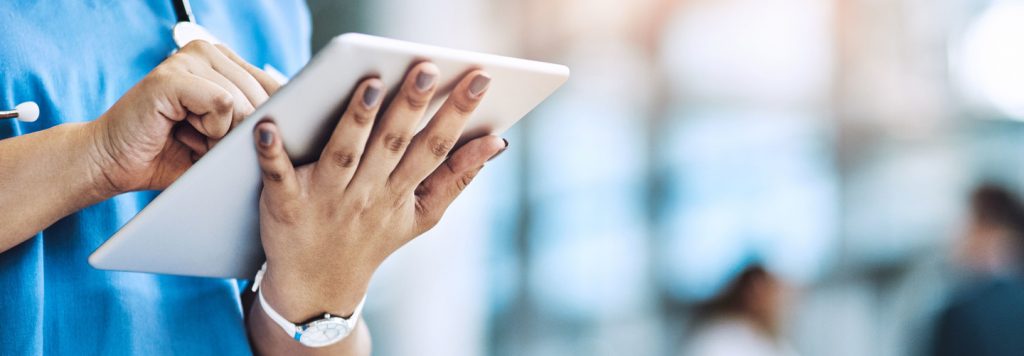
References
Bakken, J. P., Uskov, V. L., Rayala, N., Syamala, J., Shah, A., Aluri, L., & Sharma, K. (2019). Smart
education and e-learning 2018. In The Quality of Text-to-Voice and Voice-to-Text Software Systems for Smart Universities: Perceptions of College Students with Disabilities (Vol. 99). Springer International Publishing. https://doi.org/10.1007/978-3-319-92363-5_5
Brian Roemmele. (2017, January 8). The 1987 Voice First Doll: Julie by Worlds of Wonder
Commercial [Video]. YouTube. https://www.youtube.com/watch?v=ewu_NBUHePU
David, G. C., Garcia, A. C., Rawls, A. W., & Chand, D. (2009). Listening to what is said – transcribing what
is heard: the impact of speech recognition technology (SRT) on the practice of medical transcription (MT). Sociology of Health &Amp; Illness, 31(6), 924–938. https://doi.org/10.1111/j.1467-9566.2009.01186.x
Emory, J., Teal, T., & Holloway, G. (2021). Electronic note taking technology and academic performance in
nursing students. Contemporary Nurse, 57(3–4), 235–244. https://doi.org/10.1080/10376178.2021.1997148
Hawkins, C. (2022, December 16). The best dictation software in 2023.
https://zapier.com/blog/best-text-dictation-software/
Moskvitch, K. (2022, February 24). The machines that learned to listen. BBC Future.
https://www.bbc.com/future/article/20170214-the-machines-that-learned-to-listen
Movieclips. (2011, October 27). Star Trek 4: The Voyage Home (7/10) Movie CLIP – The Miracle
Worker (1986) HD [Video]. YouTube. https://www.youtube.com/watch?v=LkqiDu1BQXY
Technologies, S. L. (2022, March 7). Speech Recognition Software: Past, Present, and Future.
Summa Linguae. https://summalinguae.com/language-technology/speech-recognition-software-history-future/
The Scottish Comedy Channel. (2014, November 12). Elevator Recognition | Burnistoun [Video].
YouTube. https://www.youtube.com/watch?v=HbDnxzrbxn4
Uskov, V. L., Bakken, J.P., Howlett, R.J., Jain, L.C., SpringerLink (Online service), & SpringerLink ebooks –
Engineering. (2018; 2017;). In Uskov V.L., Bakken J.P., Howlett R.J. And Jain L.C.(Eds.), Smart universities: Concepts, systems, and technologies. Springer International Publishing. https://doi.org/10.1007/978-3-319-59454-5
Wikipedia contributors. (2023). Turing test. Wikipedia.