-
This is the first of five intellectual productions (IPs) for this course. IPs are completed individually and worth 30% of our final grade. I chose to complete this assignment in writing form to answer the four questions provided for us. Maybe in the future I will do something more creative with the IPs but thought I’d play it safe for the initial one. Proper APA formatting would have page numbers, a title page, the first line of the paragraphs indented, and hanging indents on the references. I did not meet those APA requirements due to the constraints of this website.
-
This IP asks you to:
1. Formulate a conception of usability (based on the Issa and Isaias (2015) chapter on HCI and Usability). Use what you’ve learned about usability from that chapter—but you are not summarizing or repeating their ideas. Rather, you are setting out the idea of ‘usability’ you have put together from reading that chapter. Do NOT overly rely on quotes. And remember to use proper citation practices. If you are using text that is not your own, quote and cite it, including page numbers.
2. Then, think about what is missing from this conception, from a specifically educational perspective, and on that basis try and patch together a reasonably grounded and defensible conception of educational usability.
3. Revisit Woolgar’s (challenging but rewarding!) account of “usability gone wrong,” which demonstrates several ways a usability study ended up configuring ‘users,’ thereby undermining the usefulness of usability. Identify and discuss 2 of Woolgar’s examples.
4. Finally, discuss the two excerpts quoted at the top of this IP, that have been drawn from your readings for this unit, and discuss differences you see in these 2 positions on the uses of usability.
HINT: Before you begin (and probably a few times while you are working on this) look up what “configuring” means, especially in the context of computer science. The purpose of the question is to help you to see, understand, and be able to explain how users are configured in the usability trials Woolgar observed. Elucidating and explaining user configuration in usability trials is necessary in order to understand Woolgar’s article and, therefore, in completing this task.
IP #1: Users, Uses and Usability
1. Conception of usability
Human-Computer Interaction (HCI) focuses on the interaction between humans and computers. Usability is one aspect of HCI which is about making something easy to use to meet the needs of the intended user. Good usability means a user can use the product to achieve their goals efficiently.
Usability can refer to everyday objects such as a door handle and it can be used to refer to technology products. Product developers should aim to achieve good usability and can achieve this by following seven usability principles outlined by Issa and Isaias (2015, p. 33): learnability, flexibility, robustness, efficiency, memorability, errors, and satisfaction. Since developers and users have different understandings of each other, usability works best when the product is configured to the user like Issa and Isaias (2015) suggest, not the user being configured to the product, as illustrated in the Woolgar (1990) reading.
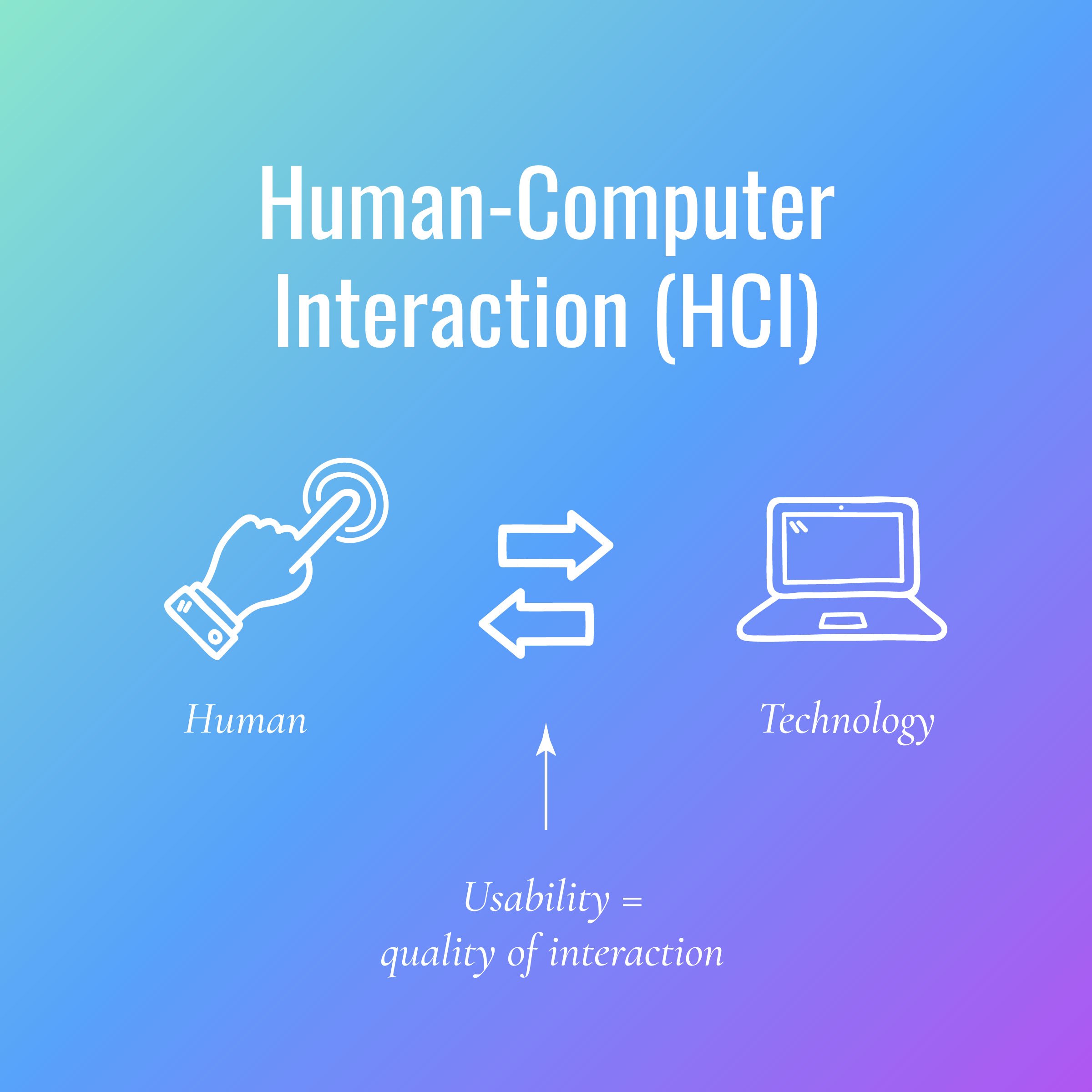
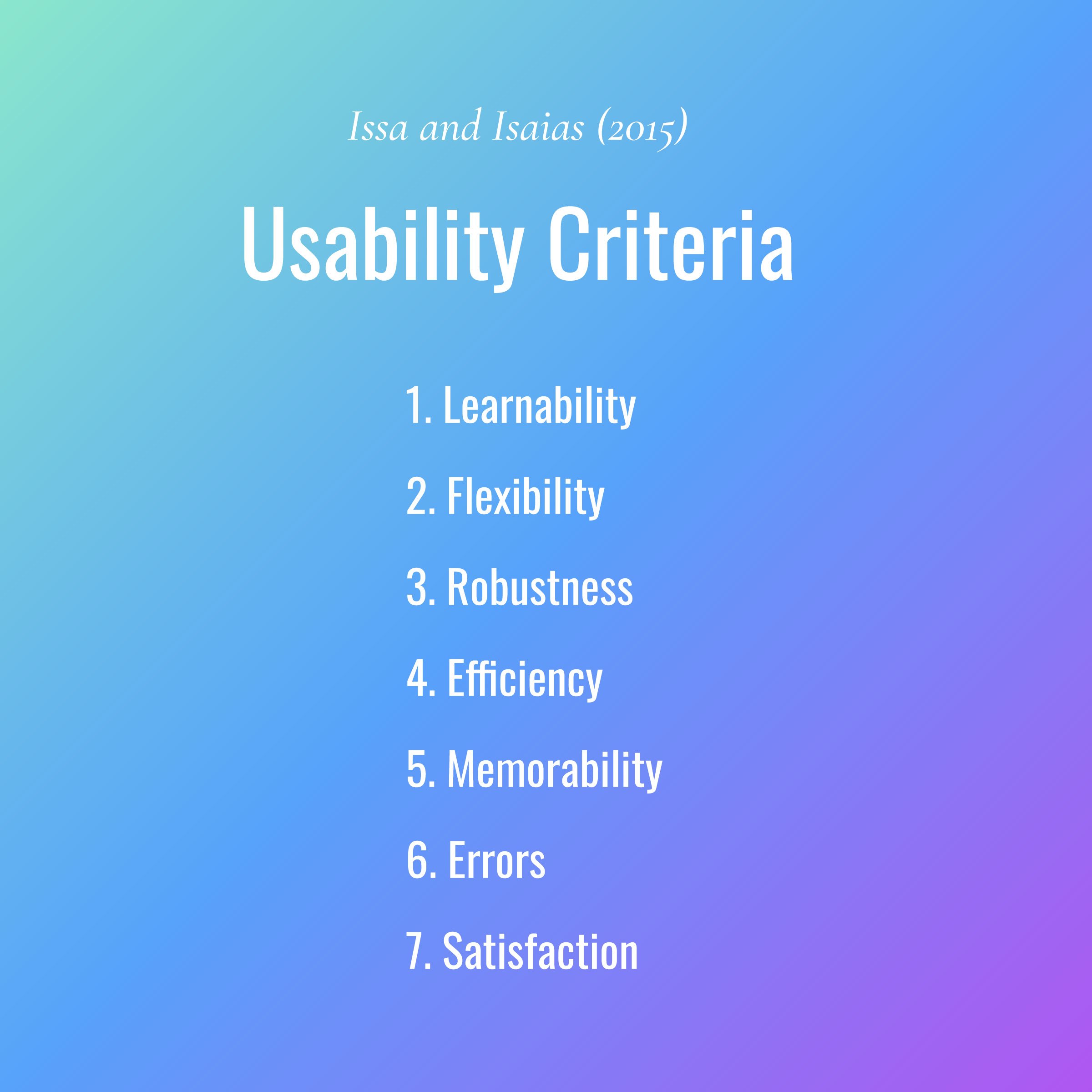
2. What is missing from this conception of usability?
Missing from this conception of usability is examining usability from an educational perspective, namely that all learners have different abilities and learning contexts.
Usability and educational usability would mostly overlap with six of the usability principles. We would critique usability (learnability), free from crashing (robustness), if the user can achieve their goals in a timely manner (efficiency), easy to remember what you were doing (memorability), have meaningful feedback when errors are made (errors), and if the user’s needs were fulfilled when using the product (satisfaction).
The principle that would look different for educational usability would be flexibility. Since each learner has different abilities, experiences, goals, and needs, what works for one student may not work for others. For example, a screen reader works great for those with visual impairments, yet is useless for someone with a hearing impairment. Educational usability describes products designed for learning where each product has its own specific learning outcomes. For learning to take place, educational usability needs to be motivating, engaging, and inclusive to all students with various abilities.
Usability and educational usability could be juxtaposed with each other since usability is about completing a task without frustration and educational usability, in some cases, can take time and can be frustrating as the learner is challenged to extend their knowledge.
When looking at usability and educational usability, we need to consider the outcomes and the affordances of the technology. Is the user engaging with the technology to complete a task for training a skill or memorization, or is the user engaging with the technology for education to develop higher-order thinking skills such as problem-solving, evaluating, or synthesizing? In addition, learning is not always directly observable or measurable so the usability of educational products could be challenging to measure as what works for one student may not work for another.
3. Usability gone wrong
Woolgar (1990) is an example of poor usability. First, the people conducting the study thought they could configure the user by having them follow instructions and manuals to complete the tasks instead of letting them be self-directed to explore and learn. The users were given prompts, hints, comments, or redirected which undermines the usability process that would happen in the natural world. If these users were learning the machines for the first time, they would most likely not have someone guiding them through this process.
Second, the selection criteria for the usability testers was poor. They wanted to find subjects “who are most likely to act as users” (Woolgar, 1990, p. 75) for the trials, which is a common problem for user testing; however, the subjects selected probably were not “true novices” (Woolgar, 1990, p. 70) since they were already familiar with the product and it would be difficult to act as an outsider.
4. Positions on the uses of usability
The first excerpt from Issa and Isaias (2015, p. 29) places the user at the centre of usability. The product developer changes the product based on the needs of the user. Issa and Isaias (2015) advocate for a user-centred approach that would translate to a learner-centred approach if referring to educational usability.
The second excerpt from Woolgar (1990, p. 59), the product is the centre of usability. The product developer believes they can configure the user to interact with the product in a prescribed way. Woolgar (1990) believes the user should adapt to the machine.
The primary difference between the two positions on usability is the perception of the user’s role. If we return to the definition of usability, usability is about meeting the needs of the user. Issa and Isaias (2015) consider the users’ perspective when developing effective tools, contrasted with Woolgar’s (1990) perspective of developing the tools first, then having the users constrained by boundaries placed on them.
References
Issa, T., & Isaias, P. (2015). Usability and human computer interaction (HCI). In Sustainable Design (pp. 19-35). Springer.
Woolgar, S. (1990). Configuring the user: The case of usability trials. The Sociological Review, 38(1, Suppl.), S58-S99.