Learning objectives
This post is about topics explored in the fifth GIS laboratory session, which had the following learning objectives:
- Learn how to independently acquire spatial datasets online;
- Parse and filter data based on your analytical objectives;
- Evaluate and use different spatial analysis tools, based on your objectives;
- Further develop your cartographic and written communication skills by producing a map and short memo summarizing your results for a non-technical audience.
The “Garibaldi at Squamish” project
The “Garibaldi at Squamish” project is proposed development of a year-round mountain resort on Brohm Ride, 15 km north of Squamish on Highway 99. An application for the approval of this project was first submitted by Northland Properties and Aquilini Investment Group of Vancouver (“Northland and Aquilini”) to the Government of British Columbia (B.C. government) in 1997 under the Environmental Assessment Act.
Following a series of addendums (additions) to the application submitted, the B.C. Environmental Assessment Office released a report in 2010 describing the lack of information on the potential environmental impacts of the proposed project and recommended several measures to prevent or reduce any significant environmental, social, economic, heritage and health effects. In April 2015, Northland and Aquilini submitted a supplemental application which they claimed addressed these issues brought up by the B.C. Environmental Assessment Office.
If approved, Garibaldi at Squamish will include 124 ski trails, 23 lifts, resort accommodation and commercial developments. It is expected to provide 900 jobs during its construction and 3,000 seasonal jobs during its operation.
There was a two month community consultation in May and June 2015, during which the Resort Municipality of Whistler submitted a 14-page letter opposing the project. It cited economic and environmental concerns and the practical viability of the project with skiing on areas of elevation less than 555 metres.
My task as a GIS analyst
In this laboratory session, I was given a scenario whereby I am a natural resource planner tasked by the British Columbia Snowmobile Federation (BCSF) to examine the report and recommendations of the B.C. Environmental Assessment Office report and the concerns of the Resort Municipality of Whistler. I am to evaluate whether there is sufficient evidence to continue to oppose the project, or whether the concerns can be addressed as part of the project.
To carry out my task, I conducted a geospatial analysis of the environmental conditions at project area using the Geographical Information System (GIS) programme, ArcGIS. This was done through the following seven steps:
1. Acquire – Obtaining data:
- I acquired data required for the geospatial analysis: ungulate winter range, old growth management areas, terrestrial ecosystems, elevation, contours, roads, rivers, project boundaries, and park boundaries.
- The datasets for ungulate winter range and old growth management areas were obtained from the DataBC website, which is a public database managed by the provincial government of British Columbia. As for the rest of the data, they were obtained from the Department of Geography, University of British Columbia.
2. Parse – Organizing data by structuring and categorizing them:
- Through the use of ArcCatalog, a geodatabase was created as the location to store all project datasets and analysis. The datasets acquired in the previous step were imported into the geodatabase.
- A file naming convention was created and all datasets and layers were named according to this convention.
- The datasets were also checked for uniformity in geographic coordinate systems and projected coordinate systems. All datasets were standardized to the GCS North American 1983 for the geographic coordinate system, and NAD 83 UTM Zone 10 for the projected coordinate system by changing the data frame’s properties.
3. Filter – Removing all data except for the data of interest:
- Some of the datasets had data that extended beyond the project boundaries. Using ArcMap, the data was restricted to within the project boundaries through the use of the “Clip” tool for both the raster and vector datasets. The clipped datasets were exported as separate files so as to retain the original datasets in case they are needed.
4. Mine – Analysis of datasets to obtain more information:
- The datasets were further processed in order to perform basic statistical analysis on them.
- The old growth management areas dataset and ungulate winter range dataset required no further processing and each dataset had their total area calculated as a percentage of the total project areas.
- The Digital Elevation Model (DEM) raster dataset containing data about the elevation of the project area was reclassified into two classes: “elevation < 555 m” and “elevation > 555 m”. The layer was then converted into polygons so that the area of elevation < 555 m can be calculated as a percentage of the total project area.
- The Terrestrial Ecosystem Mapping (TEM) layer contains data about common red-listed ecosystems in the project area. Red-listed ecosystems likely to be affected by the planned mountain resort were selected based on biogeoclimatic conditions and soil moisture and nutrient regime conditions that were similar to the project area, their total areas summed and calculated as a percentage of the total project area.
- The TRIM dataset contains data about riparian management zones and fish habitats. A multi-width buffer of protected area was created around the streams in the project area. Streams above 555 m are considered less likely to be fish-bearing and given a buffer of 50 while streams below 555 m are considered more likely to be fish-bearing and given a buffer of 100 m. These buffers were merged using the “Dissolve” tool, and the area calculated as a percentage of the total project area.
5. Represent – Choosing a basic visual model:
- The datasets were rearranged, their symbology edited, and represented on a map with a legend, title, scale, information on the coordinate system, and data source.
The general results were:
- 74% of the project area are protected areas because they were either old growth management areas, ungulate winter range, sensitive fish habitat or red-listed ecosystems. Developing the resort on any of these areas would directly impact the wildlife in these areas.
- 9% of the project area are 555 m in elevation and below, indicating that there may potentially be insufficient snow outside of winter to support the resort activities all year-round. Taking into account climate change and global warming, the minimum elevation required for year-round snowfall could decrease below 555 m and annual snowfall could also decrease year-on-year, reducing the amount of snow that will fall even during winter.
The following figure shows environmental assessment of the “Garibaldi at Squamish” project location.
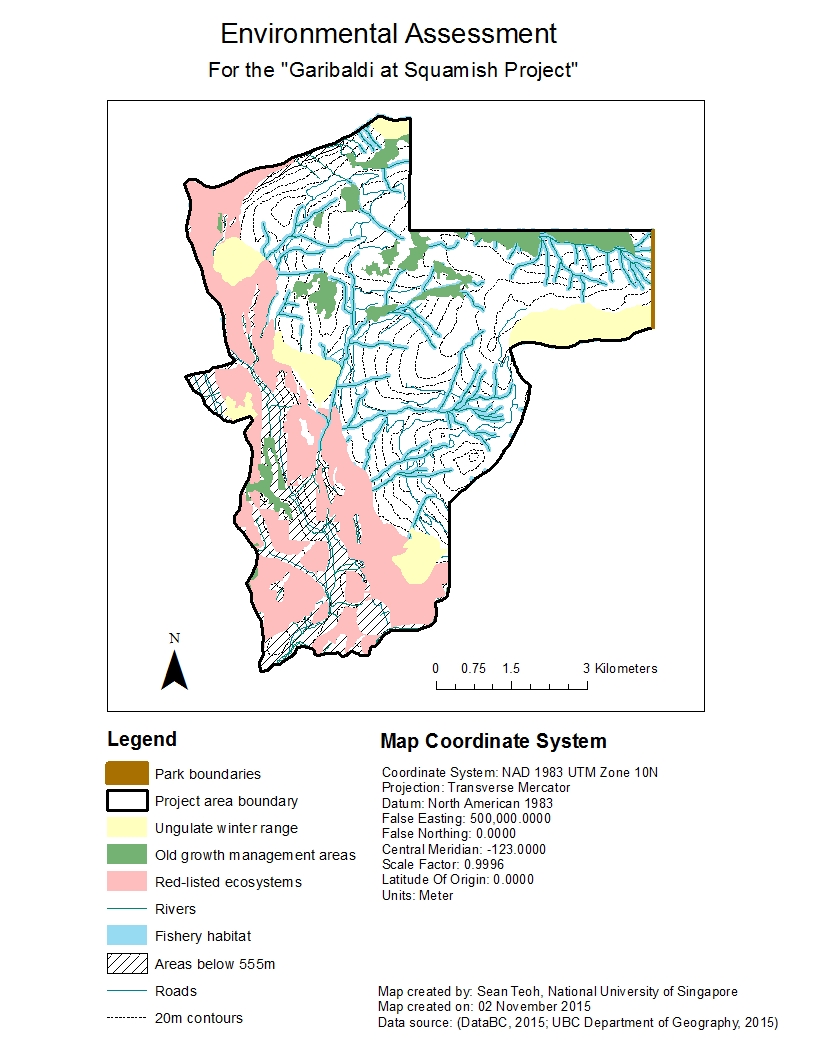
Figure 1 – Environmental assessment of the “Garibaldi at Squamish” project location.
As the red-listed ecosystems and ungulate winter range are found more at the lower elevations and around the borders of the project area at higher elevations, the two greatest environmental concerns to project development are direct impacts to fishery habitats and old growth management areas.
- If the project is to be developed on the northern part of the project area with elevation > 555 m, it will impact both old growth forests and fish habitats. Considering that only 1% of total land area in B.C. is covered by old growth forests, destroying any old growth management areas will have dire implications on the state of biodiversity of B.C. To mitigate this, the project could be developed on the southern part of the project area with elevation > 555 m, where it will impact only fish habitats. Another way to mitigate impacts on them would be to implement buffers and setbacks from the old growth management areas where no development or urban structures can be built. Fences could be built to prevent people from entering old growth management areas and causing damage.
- The Fish Protection Act under Canadian law provides provincial governments with legal power to protect riparian areas. As protecting riparian areas while facilitating urban development that embraces high standards of environmental stewardship is a priority of the B.C. government, mitigating direct impacts on these fish habitats will require more detailed environmental impact assessments by collecting data about the ecology and biology of the fish and other aquatic organisms that breed or live in these rivers, and the potential consequences if they were to be impacted. Other ways to mitigate impacts on these fish habitats would be to incorporate these natural rivers as part of the mountain resort and not drain them or develop over them. However, doing this may require the project developers to design the resort differently from initially planned.
My personal take on this project
Personally, I feel that this project should not be allowed to continue. Doing a quick check online, I found that there were already around 40 ski resorts in British Columbia alone. A study on the demand and supply of all services provided by ski resorts in British Columbia should be conducted first. If there is evidence that the supply for such services outstrip total demand, and that the supply of these services can also cater to future growth in demand, there is no strong justification to build new ski resorts. Also, if new proposed ski resorts are not substantially different from existing ski resorts (i.e. there is no novelty factor), any new ski resorts built will seem to be simply “replicas” of existing ski resorts and would thus result in only a marginal increase in the value of British Columbia as a province for skiing.
Even if we assume that there is greater demand for ski resorts than available supply and there is thus a need to increase the number of ski resorts in British Columbia, building a resort in Squamish does not make sense in terms of urban planning. This is because there is already a ski resort nearby at Whistler. Any new ski resort should be built somewhere where ski resorts are relatively inaccessible to the population nearby, so that accessibility to ski resorts would improve within the province from a macro perspective.
Also, there is a large area of riparian habitats and old-growth forests on what I imagine to be the best areas to build the ski resort on. Old-growth forests currently cover only 1% of total land area in British Columbia. While they are not protected by law (yet), they form a very important part of the ecological diversity in British Columbia, and should be conserved as long as possible. Riparian habitats, on the other hand, are protected by law; the B.C. government needs to be thoroughly evaluate this proposal because if approval is given for this project to proceed, it could set a dangerous precedent for future ski resorts to be built on other mountains with riparian habitats.