Canada and United States are founded on the same constitutional structure of the Rule of Law where laws apply to everyone equally – justice is supposed to be blind. The laws should not unfairly target or be advantageous to one person or group over another. Therefore, when I undertook the simulation, “Detain/Release,” my full intent was to make the high stake judgements in an impartial, consistent way. I was equally aware of the need to prevent overcrowding for those remanded to custody while awaiting the trial. I was also mindful that the American judicial system balances accountability to popular and political pressures with its laws. Moreover, I intended to defer to the Canadian viewpoint where Canadian and American values differed, such as drug use and guns.
Recalled
Unfortunately, I did not meet the needs of the American system. I was recalled after judging 21 defendants out of 24 due to the increase in public fear. Five of the high-risk-of-flight defendants that I had released did not return to court. Most importantly, one defendant was charged with fraud, with low risk for fleeing and risk of violence, and whom the prosecutor recommended releasing and thus I released her. Later she had a warrant out for manslaughter. This defendant caused a significant spike in the public’s confidence in my judgment.
I was consistent when reflecting on my decisions: I considered the prosecutors’ recommendations, but downplayed the defendants’ impact statement. I found myself thinking when dismissing their impact statements that perhaps the defendants should have considered their reasons before committing the crime. I had already judged them guilty, contrary to the presumptions of innocence and the guarantee of a fair process by the independent and impartial trier of facts as laid out in the Canadian Charter of Rights and Freedoms.
When I looked at the three algorithmic derived scores, I automatically released those who scored low for all three categories and found that I was not too concerned by the high-flight risk or drug use. As consistently, I detained those with a high risk for violence and took more time over the consideration about the unlawful use of weapons.
I had several questions that I pondered about: What was meant by low, medium, and high-risk? What did the system mean by “a new violent incident” when the defendant was a no-show. Would I run out of space in the detainment centre? How could you possibly predict a fraudster would kill someone?
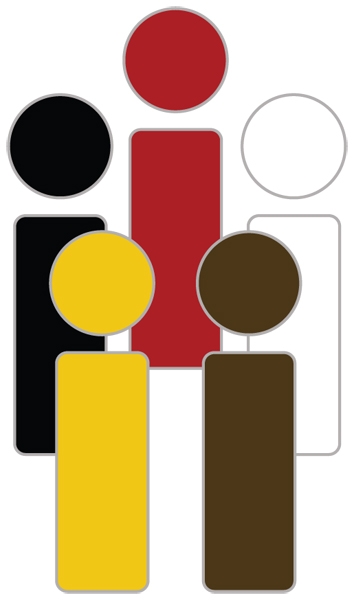
Algorithmic pretrial risk assessments are an important case study in the use of AI and algorithms in criminal justice. Bail proceedings adjudicate and balance fundamental liberty and public safety issues while needing to ensure high standards of due process, accountability and transparency”
– Law Commission of Ontario (LCO)
Pretrial Risk Assessment
Many American judicial structures introduced algorithmic pretrial risk assessment tools to reform the wealth-based bail system. It was thought that algorithms would eliminate variability, bias, and subjectivism that pervaded the courts. In addition, it was believed that the predictive algorithm would provide consistent neutral scores based on evidence to determine the defendant’s future behaviour (LCO, 2020). For example, the algorithmic pretrial risk assessment tool scores a defendant from low to high on their risk of flight, the likelihood of committing another crime, and their propensity to violence. As a result, public safety was factored into consideration.
“What qualifies as low or high depends on the thresholds set by tool designers and merely denotes the risk a group presents relative to other risk bins”
(Buskey & Woods)
The mathematical equations are trained with vast inputs of curated data from the past. Their strength is their dexterity in crunching, sorting, and evaluating the data to recognize past patterns and then propagating them to predict future behaviour (Mars, 2017; McRaney, 2018; O’Neil, 2016; 2017). Their output feedback into the system to inform future operations. To optimize the algorithm, the designer imposes a definition of success. Without mindfulness of what success is being sought, the algorithm will strongly reinforce the status quo and perpetuate any patterns of unfairness or discrimination. Mars (2017) points out that algorithms impact our society profoundly and imperceptibly.
These are not clear mathematical expressions of the way of the world… we are translating human languange, human perspective into machine language and machine perspective. As we do that we need to be careful on a meta level about how we train the system. –Allistor Croll
O’Neil (2016) argues that a good assessment tool needs to be checked and verified. She adds that the initial action is to build a fair model and then use the learning algorithm for auditing for equity, thereby increasing trust in the equations. The problem is fair for whom – this is a subjective decision. O’Neil advocates that problems in algorithms can be corrected by measurement and transparency, but when measuring the general implications, it is vital to measure for whom the algorithm injures and look at what injuries are being caused (Mars, 2017; O’Neil, 2016).
Vallor (2018) points out that artificial intelligence amplifies and extends human cognition; it augments our performance in ways that would be impossible solo. However, it does not replace human decisions for complex, unpredictable real-world problems but for simple repetitive and routine tasks that can be automated.
Perhaps there lies the problem – humans use algorithms for complex real-world situations around today’s social issues that split society. The algorithms must make a judgment call with vast amounts of bias data from the past, yet culture and values have evolved. With little or no human oversight, the system creates a feedback loop the reinforces the status quo without any deprejudicing.
“When are machines are wrong, … they are not wrong statistically. The data that we feed them is statistically more likely, But if you are trying to change that, but if you are trying to progress away from that, if you are trying to move away from the past, then this sort of bias and prejudice is a real problem It means our machines are morally wrong; they are sociall wrong, and that kind of wrongness is difficult to program out of the algorithms that we have created.” –Allistor Croll
How does this apply to Canada?
Algorithmic tools are shrouded in secrecy from how they code to what is coded. (Mars, 2017; McRaney, 2018; ) However, a fundamental to transparency and accountability of these tools would be an informed citizenry with access to open, understandable software that their government employs in the judicial, services, and policing structures (LCO, 2020; Mars, 2017, McRaney, 2018, O’Neil, 2016, 2017).
Nevertheless, there are no central lists or academic studies on how widespread predictive algorithms are in Canada, even though predictive algorithmic are already employed in numerous Canadian cities by the police service (Robertson, 2020).
“These systems are often disclosed as a result of litigation, freedom of information requests, press reports or review of government procurement websites” – Law Commission of Ontario
The increased use of algorithms in the criminal justice system for “pre-trial, sentencing, and post-sentencing phases” raises many legal issues in Canada. According to Christian (2020), there are three main issues: algorithmic racism, the legality of using AI risk assessments when sentencing, and proprietary vs individual’s Charter of Rights.
Despite Canadians pride in being a country of peace, order, and good government with a multicultural population, Canada has a long history of strained racial relations with several minority communities. For example, many police jurisdictions have been scrutinized for systematic racism and discrimination throughout the years, especially from women, black, and Indigenous groups. Thus, the Stonechild inquiry provides a poignant benchmark to gauge progress. However, unfortunately, Stonechild died during his “starlight tour’ when the city police dumped him on the city’s outskirts in the throes of winter. A common enough practice directed towards Indigenous men by some prairie city police officers. So it would be expected that if the Canadian society wished to move away from our difficult path towards a future of improved racial relations, algorithms based on the past would be less than helpful. Christian (2020) argues that the Canadian criminal justice system had an obligation to ensure that the information they use does not directly or indirectly stereotype and discriminate.
Algorithmic racism … arises from the use of historial data in training AI risk assessment tools. This has the tendency to perpetuate historical bias which are replicated in the risk assessment by these AI tools.
–Gideon Christian
Moreover, Christian (2020) quotes Justice Nakatsuru of the Ontario Superior Court, who noted that “sentencing is an individual process” and not a construct of a group membership. However, algorithmic predictive tools’ risk scores are based on statistics from analyzing big data, anything but individual. Christian (2020) supports his argument with the Ewert v Canada 2018 case in which an Indigenous defendant challenged his risk assessment because he was trained principally on non-Indigenous specifics. The Canadian Supreme Court ruled that algorithmic tools trained on one predominant cultural group are unrepresentative.
In Canada, an individual has the right to due process, an open court where they face their accuser to mount a defence against the charges; that becomes impossible to defend against the reasoning of the “blackbox” that has morphed several times since its creation or if proprietary rights trump my rights.
In Canada, an individual has the right to due process, an open court where they face their accuser to mount a defence against the charges; that becomes impossible to defend against the reasoning of the “blackbox” that has morphed several times since its creation or if proprietary rights trump my rights.
We have the freedoms to disagree with the government, the majority and work towards change. Even though Canadian society is a work in progress, and there is still far to be inclusive, what exists comes from individuals fighting for their values. However, there is no guarantee for longevity: laws, rights, and freedoms are human constructs. What would a machine construct look like?
References
Buskey, B., & Woods, A. (2018). Making sense of pretrial risk assessments. The Champion, June. https://www.nacdl.org/Article/June2018-MakingSenseofPretrialRiskAsses
Christain, G. (2020). Artificial intelligence, algorithmic racism and the Canadian criminal justice system. SLWA: Canada’s Online Legal Magazine. http://www.slaw.ca/2020/10/26/artificial-intelligence-algorithmic-racism-and-the-canadian-criminal-justice-system/
Law Commission of Ontario. (2020). The rise and fall of AI and algorithms in American criminal justice: Lessons for Canada (pp. 1–55). https://www.lco-cdo.org/wp-content/uploads/2020/10/Criminal-AI-Paper-Final-Oct-28-2020.pdf
Mars, R. (2017, September 5). The Age of the Algorithm (No. 274). https://soundcloud.com/roman-mars/274-the-age-of-the-algorithm
McRaney, D. (2018, November 21). Machine bias (No. 140). https://youarenotsosmart.com/2018/11/21/yanss-140-how-we-uploaded-our-biases-into-our-machines-and-what-we-can-do-about-it/
O’Neil, C. (2016, September 1). How algorithms rule our working lives. The Guardian. https://www.theguardian.com/science/2016/sep/01/how-algorithms-rule-our-working-lives
O’Neil, C. (2016, November 2). Weapons of math destruction. https://youtu.be/TQHs8SA1qpk
Robertson, K., Khoo, C., & Song, Y. (2020). To surveil and predict: A human rights analysis of algorithmic policing in Canada (Transparency and Accountability, pp. 1–192) [Research]. The University of Toronto. https://citizenlab.ca/wp-content/uploads/2020/09/To-Surveil-and-Predict.pdf
Vallor, S. (2018, November 6). Lesson from the AI mirror. https://youtu.be/40UbpSoYN4k