Artificial Intelligence Versus Critical Human Thinking
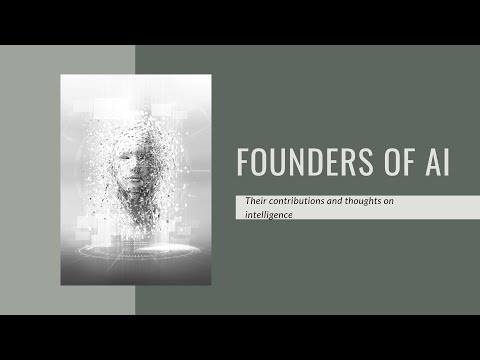
Click on the video above to watch and learn about the Founders of AI
Communication – the same, but different
Both machine and human language can fully communicate your intentions (Gurskas, 2018). Machine languages are artificial creations that do not allow you to be ambiguous; their rules and definitions were designed beforehand and do not change depending on the context, allowing for them to be fully described and studied entirely (Gurskas, 2018, Harris, 2018). Human language incorporates logical and emotional messages via words, facial expressions, body language, tone of voice, and other non-verbal cues (Harris, 2018). Unlike programming language, human language can evolve by including slang, jargon, typos, etc. (Harris, 2018).
The intelligence training ground
“Intelligence measures an agent’s ability to achieve goals in a wide range of environments.” Legg and Hutter, 2007
Human intelligence affords the capacity to approach new skills by learning and understanding different incidents and past experiences (Iyer, 2021). The human mind is a flexible and adaptable organ that turns experience into behaviour, knowledge, and skills (Chollet, 2019, p. 6). Conversely, machine intelligence is limited to generalizing the specific data sets it was trained on and cannot think independently (Chollet, 2019, p. 3-5).
Generating knowledge
Human learning can vary from person to person, especially when considering sex, age, culture, and neurodiversity; however, it is a relatively permanent change in behaviour that results from experience (Cherry, 202 ). Machine learning allows software applications to become more accurate at predicting outcomes without being explicitly programmed to do so (Burns, n.d ). This involves exposing a computer to data, and the computer then learns to make judgments, or predictions, about the information it processes based on the patterns it notices (Heilweil, 202 ). While humans and machines can discriminate in harmful ways, machine learning can “adversely affect the people who are rarely in positions to develop technology” (Buolamwini, 2019).
Transformative thinking or copy and paste?
Despite enormous progress in machine learning, artificial neural networks still lag behind brains in their ability to generalize to new situations (Sinz et al., 2019). Moravec (1988) stated it is comparatively easy to make computers exhibit adult-level performance on intelligence tests or playing checkers and difficult or impossible to give them the skills of a one-year-old regarding perception and mobility. Humans have impressive generalization capabilities due to a lifetime of diverse visual experience with natural scene statistics and generations of ancestors (Sinz et al., 2019). Further, stories bring facts to life, make the abstract concrete and, through meaning-making, walk t e listener through the mind of the scientist or mathematician (Lobato et al., 2005).
Due to the limited word count allowed for each response, there is minimal narrative and virtually no use of metaphors, similes, comparisons, or stories. While the content shared could be summarized as being a collection of facts – something that could be complied with by AI – my responses to each question went beyond generalization. In reviewing the numerous references provided as part of the module and finding through my own research, my answers were developed to facilitate a way that I could derive meaning and deep learning.
References:
Buolamwini, J. (2019, February 7). Artificial intelligence has a problem ith gender and racial bias. Here’s how to solve it. Time. Retrieved September 25, 2022, from https://time.com/5520558/artificial-intelligence-racial-gender-bias/
Burns, E. (n.d.). Machine learning. TechTarget. Retrieved September 25, 2022, from https://www.techtarget.com/searchenterpriseai/definition/machine-learning-ML
Chollet, F. (2019, November 5). On the measure of intelligence
Gurskas, D. (2018, June 20). Computer and Human Languages. Diessi.ca. Retrieved September 25, 2022, https://diessi.ca/blog/computer-and-human-languages/#:~:text=Apart%20from%20that%2C%20a%20programming,the%20only%20one%20doing%20it.
Harris, A. (2018, November 1). Human languages vs. programming languages. Medium. https://medium.com/@anaharris/human-languages-vs-programming-languages-c89410f13252
Heilweil, R. (2020, February 18). Why algorithms can be racist and sexist. A computer can make a decision faster. That doesn’t make it fair. Vox. Retrieved September 25, 2022, from https://www.vox.com/recode/2020/2/18/21121286/algorithms-bias-discrimination-facial-recognition-transparency
Iyer, A. (2021, December 8). Artificial intelligence versus human intelligence. Aretove. Retrieved September 24, 2022, from https://www.aretove.com/artificial-intelligence-versus-human-intelligence
Lobato, J., Clarke, D., & Ellis, A. B. (2005). Initiating and Eliciting in Teaching: A Reformulation of Telling. Journal for Research in Mathematics Education, 36(2), 101–136. http://www.jstor.org/stable/30034827
Moravec, H. P. (1988). Mind children: The future of robot and human intelligence. Harvard University Press.
Sinz, F. H., Pitkow, X., Reimer, J., Bethge, M., & Tolias, A. S. (2019). Engineering a less artificial intelligence. Neuron (Cambridge, Mass.), 103(6), 967-979. https://doi.org/10.1016/j.neuron.2019.08.034