The ability for scientist to develop new drugs for everything from rare diseases to headaches is often reliant on precedent and systematic investigations. These methods are often costly and time consuming. Similar problems arise in development of new materials that may enhance our energy production. Our limited ability to rationally design materials hampers their development. This leads to reliance on our ability to recognize the trends and behavior of already existing materials. However, what if we could amplify the ability to recognize patterns beyond human limits? Machine learning answers this problem.

A graph depicting the general algorithm machine learning follows. Source: Wikimedia Commons
While machine learning is a form artificial intelligence, our jobs are safe. Machine learning is the use of statistics and the power of computers to predict results or identify trends in data. The general method relies on the input of “training” data which is analyzed using statistics. After developing a model, information may be inferred from new data the computer encounters.
-Video Source: Google Cloud Platform educational AI Adventures Series on YouTube by Yufeng Guo in 2017.
Large technology companies have recognized the advantage of integrating machine learning into technology development. Google is one example that has successfully introduced it. Gmail uses machine learning to service 1.5 billion active accounts. They claim to detect 99.9% of phishing and spam mail from entering the user’s inbox. However, machine learning is not limited to technology companies. Chemistry researchers have quickly adopted it.
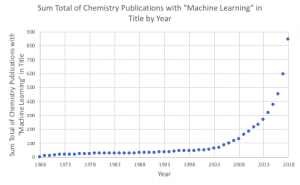
Total Number of Chemistry Publications with “Machine Learning” in Title
Starting in 1969, the first chemistry journal article with “machine learning” in the title was published. By combining machine learning with a common technique called mass spectrometry, Peter Jurs at the University of Washington was able to determine chemical composition of “unknown” chemicals using the input of 348 unique patterns as “training” data.
More recently there has been an almost exponential increase in the number of chemistry publications applying machine learning. In the last two years approximately 6 times as many publications were made than in the past 48 years. Tommi Jaakkola, a Professor of Electrical Engineering and Computer Science at MIT said at a consortium about implementing machine learning in the pharmaceutical industry: “by marrying chemical insights with modern machine learning concepts and methods, we are opening new avenues for designing, understanding, optimizing, and synthesizing drugs.” The materials science community has also seen integration with the development of novel long chained molecules called polymers for photovoltaics by scientist at Osaka University. Shinji Nagasawa, the lead author explained the importance: “there’s no easy way to design polymers with improved properties. Traditional chemical knowledge isn’t enough. Instead, we used artificial intelligence to guide the design process.”

Solar cell efficiency over years showing a substantial increase. Source: Wikimedia Commons
While machine learning is not the solution to all chemical problems or spam mail, it is being widely accepted by the scientific community and technology industry for good reasons. Even with limitations, it’s effectiveness across a wide array of industry and research emphasizes the role it may play in the future of research and development.
—Jonah
References
- Graph-powerd Machine Learning at Google. Google AI Blog. https://ai.googleblog.com/2016/10/graph-powered-machine-learning-at-google.html (Accessed Feb 28, 2019).
- Jurs, P.C.; Kowalski, B.R.; Isenhour, T.L. Computerized Learning Machines Applied to Chemical Problems: Molecular Formula Determination From Low Resolution Mass Spectrometry. Chem. 1967, 41, 21-27.
- Machine Learning, Materials Science and the New Imperial MOOC. Imperial College London. https://www.imperial.ac.uk/news/187054/machine-learning-materials-science-imperial-mooc/ (Accessed Feb 28, 2019).
- UBC Summons. University of British Columbia.
4 responses to “Is Machine Learning the Future of Technology Development and Chemistry Research?”